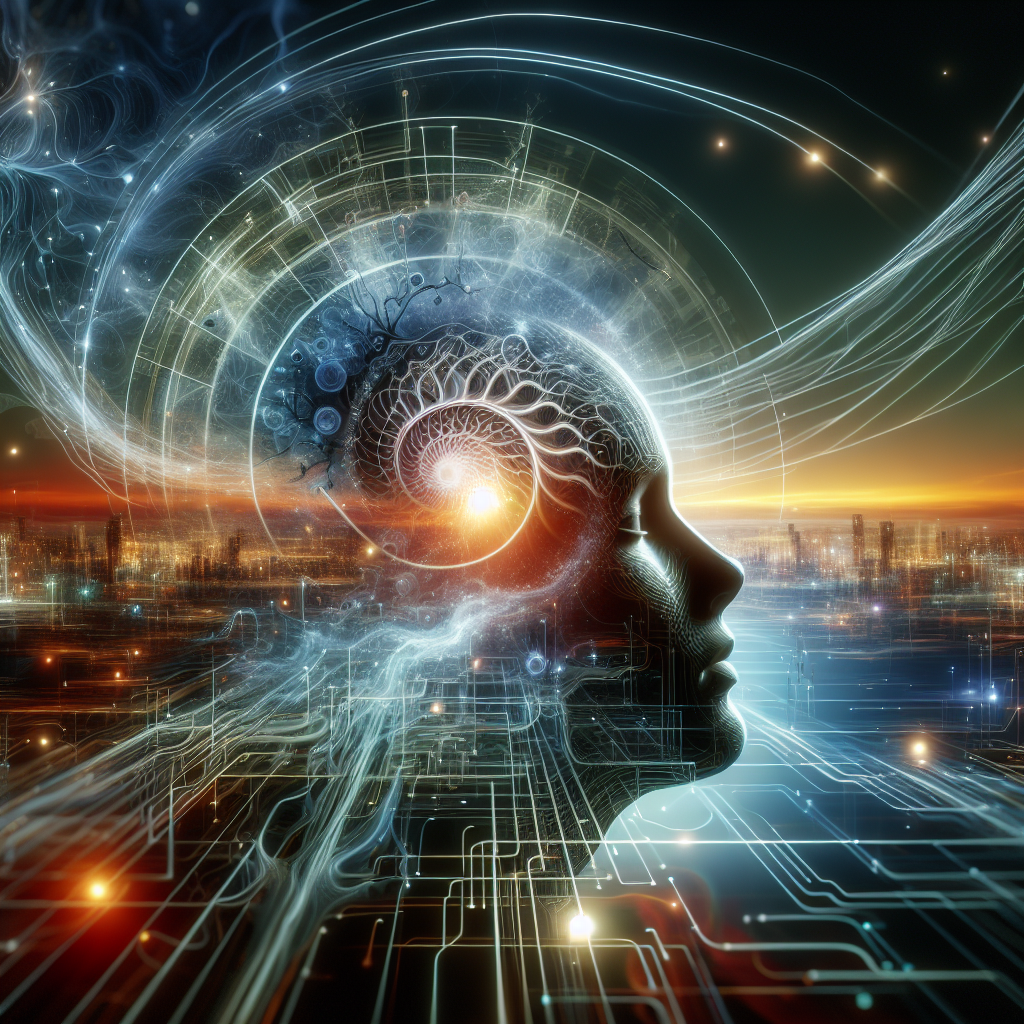
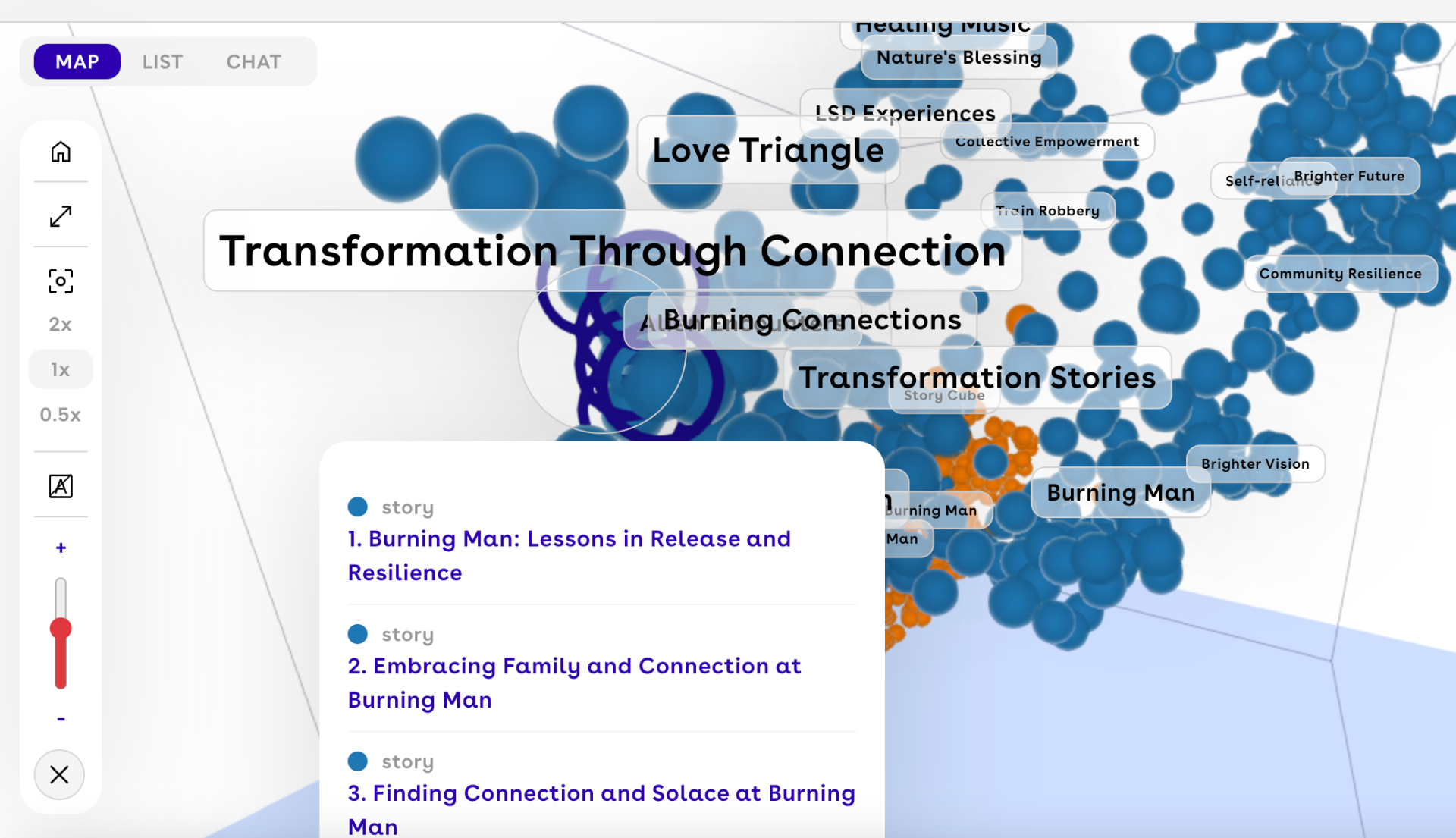
Meet the AI
explore data, chat with the AI and share your story!
The building blocks of Burner AI employ machine learning techniques to systematically analyze and visualize relationships within a diverse collection of dataset, including shared participant data of 490 stories and combine it with a indexed database of all Burning Man research with 290 research articles and 60 podcasts By integrating empirical works with published research in a singular, interactive platform, the Burning Stories project not only democratizes access to narrative insights but also contributes to the broader discourse on cultural and transformational trends. The AI platform's potential for dynamic data clustering and topic modeling aims to increase our understanding of how communities form, evolve, and invites you to share a story.
The chat function is intended to bring to the surface the hidden knowledge of these cultures by facilitating real-time interaction between users and the data. It allows participants to engage directly with the AI, ask questions about the narratives, and explore patterns within the stories. By doing so, the chat serves as both an educational tool and a storytelling medium, helping users navigate through layers of community wisdom and personal transformation experiences.
Additionally, the chat feature enables users to contribute their own insights or interpretations, fostering a participatory research environment. It encourages deeper engagement with the dataset, enhancing our collective understanding of how cultural narratives around transformation are constructed, perceived, and shared across diverse festival communities. This makes the project not only a repository of knowledge but also a living archive that grows with each interaction.
Burner AI´s technical description
The applied methodology encompasses a multifaceted approach to data collection, processing, and visualization. Initially, a corpus of narrative data—including transformational in situ stories and related scientific articles—is compiled. Data is structured for analysis, employing Natural Language Processing (NLP) techniques for summarization. Subsequently, the narratives undergo an embedding model, utilizing modelings to capture the nuanced dimensions of the data. Dimensionality reduction via Uniform Manifold Approximation and Projection (UMAP) and clustering through Hierarchical Density-Based Spatial Clustering of Applications with Noise (HDBSCAN) facilitate the organization of data into coherent, thematic clusters. This process is augmented by Term Frequency-Inverse Document Frequency (TF-IDF) analysis to highlight key terms, and Large Language Models (LLMs) to generate descriptive labels for each cluster, encapsulating the predominant themes. The outcome of this process is an interactive, visual map that positions narrative data points according to semantic similarity. This visualization not only fosters intuitive exploration of thematic areas but also encourages deeper engagement with the underlying stories. By rendering the data in two dimensions, the platform offers users a unique way to navigate the complexity of human experiences, reflecting the diversity and interconnectedness of the stories collected and existing research.